A new metric for stablecoins
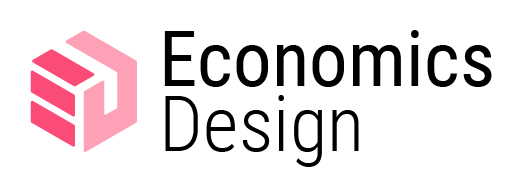
This is not financial advice.
One of the significant problems we face when analysing final risk of stablecoins is the different ways they operate, which makes it difficult to conduct comparisons across different types of stablecoins in the market. We cannot deduce if a stablecoin with on-chain collateral is better in managing financial risk exposures than an off-chain peer, or to conclude if a specific type of algorithm is better than others in maintaining price at the initially pegged value. Although the construction of token and how they operate are the building blocks of where financial risk come from, we cannot make conclusions based on such qualitative judgements only. A possible solution here is to look for a method that quantitatively describes the ability of these stablecoins in maintaining price, and here we have the price deviation metrics. In this article, we will introduce what the price deviation metric is, its distinctions from other metrics like price volatility, and show how a simple comparison can be done across different types of stablecoins using this method.
Price deviation metrics
The term price deviation here refers to how much the market price of a stablecoin is deviating away from the pegged value. For simplicity, we will take the pegged value as $1 USD here. Thus the token has positive deviation when its price is above $1, and negative if it is below $1.
Deviation = price – 1$
Comparison with standard deviation
As compared to the standard deviation method of measuring price stability, the price deviation method is more suitable for stablecoins for a few reasons. Firstly, the standard deviation measures the amount of variations around the mean in a set of values. But from empirical observations, the mean price of stablecoins may not be exactly at $1 and may be significantly away from $1depending on the stage of development, so standard deviations may give overly optimistic results on the ability of token pegging to its fixed value.
Secondly, the standard deviation treats variations in both positive and negative directions equally. This is different from the reality, where prices could be higher or lower than $1 at more of the times. It is important to recognise these trends, as they may hint that the protocol has different ability to maintain its price under different price conditions, which add to financial risk exposures.
Data required
To calculate price deviation, we need a time series of prices for the token to be analysed. Depending on the granularity of the data source, time intervals could be fixed at daily, hourly, minutely or even smaller. To get more accurate results, use smaller time intervals so movements of prices with time can be shown better. Anomalies also should not be removed for a complete testing of price pegging ability. The suggested minimum sample size to be used is 50.
Calculations of price deviation
The first step of price deviation analysis is to generate a time series of price deviations using the above formula. Then, count the number of positive and negative deviations, and calculate the descriptive statistics (mean, maximum, minimum, 25th percentile) for each group. This gives the general trends of price deviation. Below is an example of deviation results using hourly price series of FRAX for a period of 90 days from Nov 2021 to Feb 2022:

Fig 1. Example of FRAX price deviation
At this point, we can already observe some trends in the token’s price when its price goes above and below the peg. The occurrence of prices going above the peg is more than double the occurrence of prices going below. The average magnitude of deviation is also higher if it is above the peg, but negative deviation has higher maximum and 25th percentile value, indicating that price movements are greater in the more extreme situations.
Next, for all time intervals, we need to consider what is the price movement in the next interval. This price movement is calculated as the difference between prices in these two consecutive intervals.
Price movement = price(t) – price(t-1)
Under ideal situations, if current price is above $1, it should decrease in the next interval to return to the peg. If current price is below $1, it should increase. Based on this, generate a list of TRUE/FALSE indicators of whether price is moving in the right direction towards the peg in the next interval. This is the directional change of prices.

Fig 2. Directional change of FRAX
We see that price is moving in the direction back to pegged value most of the time. There is no significant difference between deviations in positive or negative too, demonstrating a good price maintenance mechanism, hence low financial risk.
Comparison of risk
The price deviation method provides a simple way of conducting financial risk analysis. We can apply the same procedure to a range of tokens using data of the same granularity and time period to make a comparison between tokens, or to apply it across different time periods of the same token to see how financial risk level may have changed over time. Below is an example for comparison of 12 different stablecoins from Nov 2021 to Feb 2022.

Fig 3. Price deviation results of 12 different stablecoins
Numbers in red represent values that indicate higher financial risk, while numbers in green represent lower financial risk. We see that for all tokens compared, the price maintaining mechanism are working well most of the times. A brief comparisons of the numbers shows that BUSD and FEI have relatively lower level of financial risk, while LUSD and UST face higher level of financial risk. Across categories, stablecoins backed with off-chain fiat collaterals also have the best ability in maintaining the price peg.
Potential problems
Despite the simplicity to use, we should also be aware of the potential issues with using the price deviation method for financial risk estimation. The most major problem is that analysis result is highly impacted by the choice of data, in both length of time period and frequency of prices used.
Using data of a shorter time frame may give overly optimistic result on the token’s ability in maintaining price at its pegged value, especially if there has not been a market shock in that time. Meanwhile, using longer time period can ensure our analysis accounts for more events and price reactions, but overly long time period that extends into different stages of the token’s development may also be less relevant, if our focus is to estimate risk in short terms.
In addition, frequency of data used is also significantly relevant to the accuracy of analysis. If we use data in a daily or hourly frequency, the price at point of capture are actually not reflecting the immediate impact of the price maintaining mechanism as there could be many price movements in between the time points.
Conclusion
This article provides a possible method for analysing financial risk exposures of stablecoins that can be applied across all categories. The stablecoin market today is actively growing with new algorithms to peg token price to a fixed value, and the wide variety of assets used as collaterals are adding to the difficulty of conducting a standardised quantitative analysis. Together with qualitative analysis on the token design, price deviations evaluates the effectiveness of these mechanisms under real market situation and allow us to understand the risk levels faced by tokens better.
Similar to all other risk analysis, the key point is to always have a clear goal in mind. In order to come up with a meaningful conclusion, time frame and interval of data should be carefully chosen to meet the goals of analysis. Significant irregularities should also be matched with market happenings, to uncover the reasons behind such events. Although market conditions are unlikely to replicate in future and we should not expect impact to be the same if such events occur again, they would provide valuable tools for future risk estimation and projection.